报告题目:Making and breaking electronic structures: lessons from embedding and machine learning
报告嘉宾:Michele Pavanello,Rutgers University 教授
报告时间:2024年9月14日 10:00
报告地点:伟德BETVlCTOR1946中心校区唐敖庆楼B521报告厅
嘉宾简介
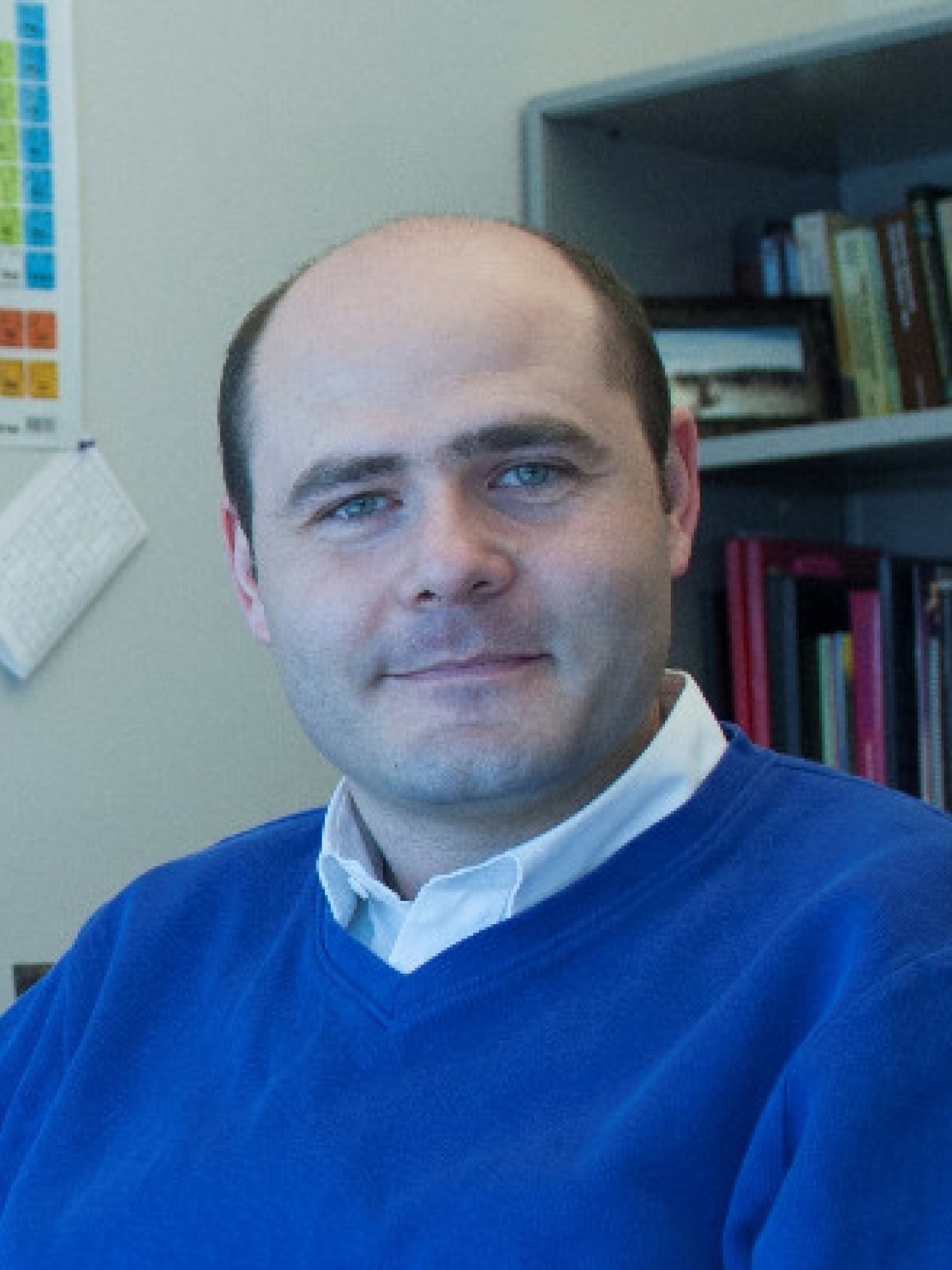
Michele Pavanello is a professor of Chemistry and Physics in the Newark campus of Rutgers University. Michele graduated in 2010 with a PhD in Chemistry from the University of Arizona working with Prof Ludwik Adamowicz on describing few-particle Coulomb systems such as the H3+ molecular ion as accurately as possible. A Marie Curie fellowship took him to the Netherlands to work on density embedding and its formulations for describing charge-transfer reactions with Prof Johannes Neugebauer (now at Munster, Germany). Since 2012, Michele has been leading the Pavanello Research Group (PRG) focusing on electronic structure method development, targeting material science as a major focus, funded by NSF, DOE and ACS-PRF grants. Michele received the ACS-COMP OpenEye junior faculty award in 2016, and in the same year he received the NSF CAREER award. In 2018 and 2020 Michele was awarded the Rutgers Board of Trustees Award for Excellence in Research for recently promoted as well as for tenured faculty. In 2022, the Physical Chemistry division of ACS awarded him the ACS-PHYS Early Career Award for Theoretical Chemistry.
报告简介
The most enthusiastic modeler claims to accurately predict chemical reaction thermodynamics, kinetics, and nonequilibrium dynamics. Unfortunately, current models, while more robust and predictive than in past years, are often either too approximate or too expensive to provide a faithful representation of reality. Thus, it is imperative to develop new-generation electronic structure methods to aid experiments, as these face different yet similarly difficult circumstances. The talk introduces models based on orbital-free density-functional theory (OF-DFT), machine learning and density embedding to tackle large molecular condensed-phase systems that are too extensive for conventional electronic structure methods. To achieve chemical accuracy, nonstandard workflows are presented. These involve the dynamic combination of OF-DFT and conventional DFT methods, resulting in black-box-like adaptive embedding methods where molecular fragments are merged and split dynamically along a Born-Oppenheimer dynamics trajectory. The presented methods are available to the broader community as open-source Python implementations, such as Quantum ESPRESSO in Python (QEpy, qepy.rutgers.edu), OF-DFT software (DFTpy, dftpy.rutgers.edu), and density embedding software (eDFTpy, edftpy.rutgers.edu). The argument is made that with such a software arsenal at our disposal, we are ready to tackle the most difficult and timely electronic structure challenges available today.
举办单位:
bv伟德国际体育
物质模拟方法与软件教育部重点实验室